NCWIT Selects 2024 AiC Collegiate Award Recipients
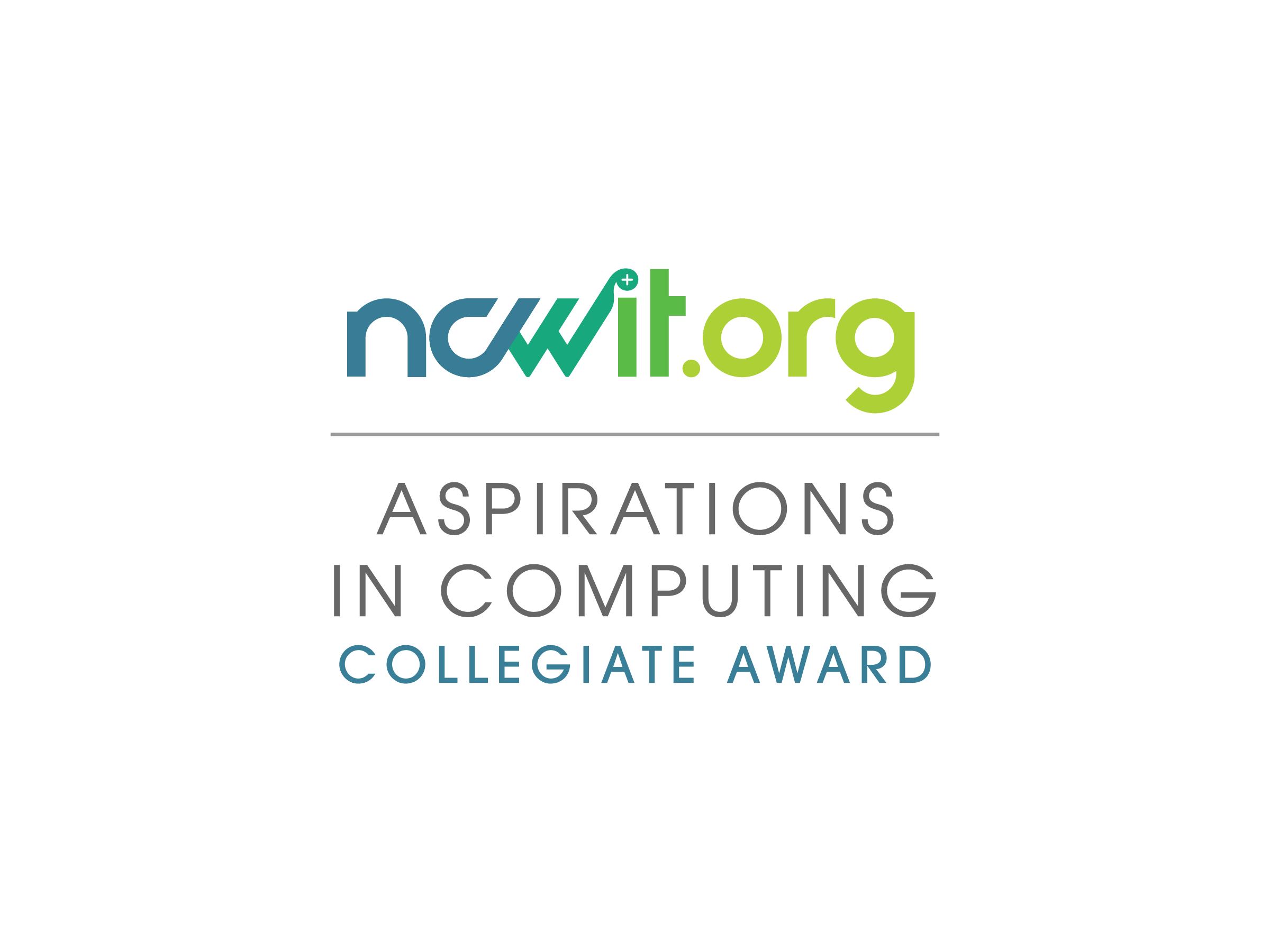
The National Center for Women & Information Technology (NCWIT) Aspirations in Computing Program (AiC) is pleased to announce the recipients of the 2024 NCWIT AiC Collegiate Award, celebrating 12 undergraduate and graduate women, genderqueer, and non-binary students from 11 academic institutions nationwide. Conferred annually, the award recognizes technical contributions to projects that demonstrate a high level of innovation and potential impact.
The multi-tiered award structure includes Winner and Honorable Mention.
The entire NCWIT AiC program platform is supported generously by Apple. AiC also receives support for specific national program elements; the NCWIT Collegiate Award is sponsored by Bank of America.
Winners
- Hannah Guan (she/her) | Class of 2027 at Harvard University
Multi-Dimensional Interpretable Interaction Network (MDiiN) for Modeling Aging Health and Mortality
Aging is a complex stochastic process that affects healthy functioning through various pathways. In contrast to the more commonly used cross-sectional methods, this research focuses on longitudinal modeling of aging, a less explored but crucial area. They have developed a Stochastic Differential Equation (SDE) model, at the forefront of aging research, designed to accurately forecast the health trajectories and survival rates of individuals. This model adeptly delineates the connections between different health indicators and provides clear, interpretable results. Their approach utilizes the SDE framework to encapsulate the inherent uncertainty in the aging process. Moreover, it incorporates a Recurrent Neural Network (RNN) to integrate past health data into future health projections. They trained and tested the model using a comprehensive dataset tailored for aging studies. Their model is not only computationally cost-effective but also highly relevant in assessing health risks in older populations, particularly for those at high risk. It can serve as an essential tool in anticipating and preparing for challenges like infectious disease outbreaks. Overall, this research aims to improve health equity and global health security significantly, offering substantial benefits to public health and deepening our understanding of the aging process. - Ryan Karim (they/them) | Class of 2024 at Arizona State University
▶️ Mind Over Matter: Improving Prosthetic Outcomes with Smart Sensors and AI
Globally, there are 100 million people in need of a prosthetic device, according to the World Health Organization. Only 1 in 10 people in need have access to assistive devices due to prohibitively high costs and lack of availability. This research project aims to develop a prosthetic arm that could dynamically change behavior based on the wearer's existing nerve impulses. They designed an approach that utilizes the body's electrical signals generated during muscle activity to control the movement of a 3D-printed battery-powered prosthetic arm. These impulses, called electromyographic (EMG) signals, were detected by noninvasive surface electrodes placed on the skin. The prosthetic arm utilized a machine learning algorithm called long short-term memory (LSTM) to analyze EMG signals and distinguish complex hand movements, increasing the range of motion and adaptiveness of the prosthetic in an accurate and reliable manner. With a total cost of just under $450, this prosthetic is an affordable, adaptive solution for patients, delivering more natural control and functionality in their daily activities. - Doreen Joseph (she/her) | Class of 2024 at the University of California, Davis
▶️ Embedded Firmware Binary Security Analysis Using Transformers
Embedded systems have become pervasive in today's world, bringing along with them a large attack surface that has proved challenging for security practitioners. To operate, these systems rely on specialized software, known as firmware, that has become a major source of security concerns in recent years. In this project, they aimed to leverage a Transformer-based embedding model equipped with instruction trace and control flow graph (CFG) structure information to learn semantically-rich vector representations of functions in firmware binaries. These embeddings could then be used for downstream tasks such as binary similarity analysis and identification of vulnerabilities present in the firmware. Control flow graphs (CFGs) are graphical representations of all the possible paths a program can take during execution, thus making them inherently complex. Given the fact that they have no canonical ordering, they leveraged a technique from spectral graph theory to capture the structural information for input into their Transformer model. They applied Laplacian eigenmaps, a state-of-the-art technique in graph theory, to create a low dimensional embedding for each function's physical control flow structure, which remarkably improved their model's statistical understanding of disassembled code as compared to a model without the additional CFG structure information. The results from their technique demonstrated that a structure-aware Transformer model notably improves the learning of firmware binary code semantics.
Honorable Mentions
Angelly Cabrera (she/they) | Class of 2024 at the University of Southern California
▶️ Early Detection of Coffee Leaf Rust through Convolutional Neural Networks: A Climate Change Adaptation Strategy
Coffee leaf rust, a disease caused by fungus, poses a major threat to coffee production, especially in Central America. Climate change has exacerbated this issue by shortening the latency periods of diseases like leaf rust, increasing the urgency for effective disease management strategies. The consequences of this are drastic, adversely affecting Central American economies, where coffee contributes to over 20% of exports. This project aims to leverage signal processing and convolutional neural networks (CNNs) to detect early signs of coffee leaf rust and help coffee farmers, especially in Guatemala's diverse coffee-growing regions, who face significant risks due to the disease. Through CNNs and signal processing, they created a non-invasive solution for early leaf rust detection by designing a high-pass filter to manipulate image data before training my model. By applying this high-pass filter, they processed images that realistically represent the conditions in which farmers typically capture photos of their crops. This approach was essential to ensure the technology could accurately identify disease symptoms even in less-than-ideal imaging conditions. Their ultimate goal is to make this technology accessible to farmers in under-resourced communities, who often face the greatest economic and environmental inequities. By providing farmers with a tool for early disease detection, they aim to offer a practical solution to mitigate the impacts of these challenges, enabling more effective and timely responses to crop diseases.
Anika Chebrolu (she/her) | Class of 2024 at the University of North Texas
▶️ DeepLig: A de-novo Computational Drug Design Approach to Generate Multi-Targeted Drugs
Mono-targeted drug discovery has paved a significant path in the history of drug design. However, more recently, these mono-targeted and highly selective drugs have proven to lack efficacy, especially in the face of complex diseases that depend on multiple receptors at once for pathogenesis, such as neurodegenerative diseases and cancers. Hence, multi-targeted drug design was introduced to create more robust drug candidates that inhibit multiple biochemical receptors at once for greater efficacy. However, the scope of current computational approaches for multi-target drug design is limited. The goal of this project was to develop DeepLig, a computational model that can successfully guide a pre-trained generative model towards the generation of multi-targeted drugs, given the input of multiple protein targets. The algorithm can be divided into two separate networks that work in interplay using reinforcement learning: a generative network and a predictive network. The generative network uses a Stack-Augmented Recurrent Neural Network (Stack-RNN) to generate novel chemical compounds, while the predictive network uses a Graph Attention Network (GAT) to predict the binding fitness of a compound towards multiple target proteins. This study proposes that DeepLig provides an effective approach to design multi-targeted drug therapies that can potentially show higher success rates during in-vitro trials.
Divya Gupta (she/her) | Class of 2027 at Carnegie Mellon University
▶️ Algorithmic Reconstruction of Damaged Dendrites to Prevent Food Contamination
The project, "Reconstruction of Damaged Dendrites to Prevent Food Contamination," aims to solve the growing issue of food contamination by using high-entropy dendritic tags on produce. What happens when these tags get damaged in the packaging process and are unable to be traced? Scratches, bumps, or a little roughhousing with other fruits, and suddenly, these tags could lose their accuracy. While this might not immediately strike as a serious issue, where one in ten people globally face foodborne illnesses yearly, even a small percentage of damaged tags could mean a big problem. What happens when there is a food outbreak, and when the time comes to trace it to its source, there are gaps in the chain? To solve this, they designed an algorithm using OpenCV and TensorFlow that can scan and patch up damaged dendrites, ensuring that every produce has a unique tag that can be traced even if it gets damaged during the packaging process. By reconstructing the original tag, their algorithm promises to trace produce back to its roots, slamming the brakes on contamination at the source.
Yuexing Hao (she/her) | Class of 2026 at Cornell University
▶️ Advancing Patient-Centered Shared Decision-Making with AI Systems for Older Adult Cancer Patients
This project addresses the vital role of shared decision-making (SDM) in cancer and its impact on enduring therapeutic communication and patient-clinician relationships. Previous research has shown that active patient participation in decision-making enhances satisfaction and treatment outcomes, yet medical decision-making is often complex. The project aims to understand the unique needs and perspectives of older adult cancer patients and their clinical providers. The central research question is whether these stakeholders perceive the patient-centered AI SDM system as a potentially effective tool for improving clinical efficiency and enhancing the healthcare experiences and outcomes of older adult cancer patients. The primary contribution of this project is the demonstration of the feasibility of optimizing the health outcomes of older adult patients through well-tailored treatment plans, increased patient engagement, and compliance. Additionally, the patient-centered AI system has the potential to elevate the healthcare experience, empowering older adult cancer patients and enhancing their knowledge of their treatment plans. Furthermore, this work contributes to the field of SDM by improving resource allocation, reducing time pressures on clinicians, and fostering stronger patient-clinician relationships.
Sirihaasa Nallamothu (she/her) | Class of 2027 at the University of Illinois Urbana-Champaign
▶️ Predicting Vasovagal Syncope in Postural Orthostatic Tachycardia Syndrome Using Machine Learning Algorithms, Wearable Sensors
Postural orthostatic tachycardia syndrome (POTS), is a rapid variability in heart rate that causes vasovagal syncope: a temporary lack of blood flow to the brain leading to fainting (outs). Patients often suffer injuries because they have no warning symptoms before collapsing. This project uses machine learning to predict syncopal episodes, ensure personal safety, and empower the POTS patient to regain control of their "outs." To create the algorithms, they decided to collect their own data sets by running a clinical research study. They gathered clinical physiological data on POTS patients using an Empatica smartwatch. They collected heart rate, blood volumetric pressure, electrodermal activity, temperature, and accelerometer data for a total of 21 days from three participants. The final data set consisted of 868 hours of sensor data with 74 tagged syncopal episodes. The final model was a multimodal late fusion model, consisting of a 1D convolutional neural network (CNN) connected to a Long Short Term Memory neural network (LSTM), which helped with the temporal prediction: the model was trained on non-invasive physiological signals. The final 1D CNN-LSTM model was trained to identify vasovagal syncope episodes with an F1 score of 0.89 and an AUC of 0.95. The model was able to predict the onset of syncope two minutes in advance with an F1 score of 0.69.
Adrianna Pinzariu (she/her) | Class of 2025 at DePaul University
SKIIP: Enhancing Campus Safety with AI-Optimized Navigation and Human Trafficking Detection
More than just an application, SKIIP rеprеsеnts a significant commitmеnt to еnhancing campus sеcurity and student safety. The app provides AI-optimizеd safе routеs for navigating thе campus, incorporating a range of data including rеal-timе updatеs and historical safеty records. This fеaturе is particularly crucial during latе-night hours, offering studеnts a safеr way to travеrsе their campus whеn thе risk of incidеnts is known to incrеasе. SKIIP's functionality еxtеnds beyond mеrе routе optimization. It includes SеcurеAI, a spеcializеd componеnt dеsignеd to tacklе thе intricatе issuе of human trafficking. SеcurеAI lеvеragеs advancеd algorithms to dеtеct pattеrns and signals that arе indicativе of trafficking activitiеs, with a particular focus on off-campus еnvironmеnts such as hotеls. This aspect of SKIIP is еssеntial for students who frеquеntly еngagе in off-campus activities, providing them with critical safety information about their surroundings. Thе opеrational mеchanism of SеcurеAI involvеs thе analysis of data from hotеl wеbsitеs. It utilizеs dееp lеarning to identify outliеr imagеs in hotеl rooms that might suggest illicit activities. In parallеl, thе systеm conducts sеntimеnt analysis on hotеl rеviеws, sеarching for nеgativе pattеrns or alarming signals that could indicatе unsafе conditions or trafficking activitiеs. This comprеhеnsivе approach еnablеs thе idеntification of potential risks associatеd with specific hotеl locations. By intеgrating SеcurеAI into SKIIP, thе application's protеctivе rеach is significantly еxpandеd beyond thе confinеs of thе campus. SKIIP, couplеd with SеcurеAI, is not mеrеly a tеchnological solution, it is a movеmеnt towards a morе sеcurе and еmpowеrеd studеnt community. It aims to rеdеfinе thе narrativе around campus sеcurity and studеnt wеll-bеing.
Sanaz Rabinia Haratbar (she/her) | Class of 2024 at Wayne State University
▶️ Data Sharing Aware Task Allocation in Edge Computing System
This project is the first systematic work to study the data sharing-aware problem on Edge Computing. They formulated the data sharing-aware allocation problem, which has the objectives of maximizing profit and minimizing network traffic by considering the data-sharing characteristics of tasks on servers. Because the problem is NP-hard, they designed the DSTA algorithm to find a feasible solution in polynomial time. They investigated the approximation guarantees of DSTA by determining the approximation ratios with respect to the total profit and the amount of total data traffic in the edge network. They analyzed the performance of the proposed algorithm against a state-of-the-art baseline that only maximizes profit. Their extensive analysis shows that DSTA leads to about 8 times lower data load on the network while being within 1.03 times of the total profit on average compared to the state-of-the-art. To the best of their knowledge, their work is the first to exploit the key intuition that, in edge systems, multiple data-driven tasks from each user device may share some data items. This intuition can be exploited to reduce the network load in edge computing systems by allocating high data-sharing tasks to the same servers.
Aarushi Sarbhai (she/her) | Class of 2024 at the University of Utah
▶️ Reactive Spectrum Sharing with Radio Dynamic Zones
The wireless radio spectrum has been divided among various users by regulatory bodies, leaving almost no unclaimed spectrum. However, there's a growing demand for spectrum access from existing users and emerging technologies. Efforts are underway to reclaim some sub-6 GHz radio frequency spectrum, allowing secondary use through dynamic spectrum access. Radio Dynamic Zones (RDZs) are an emerging concept to enable dynamic spectrum sharing with consumer broadband, special transmitters such as directed energy systems, high-power microwave transmitters, and other experimental radio systems. They developed a scalable, novel reactive Zone Management System (ZMS) for spectrum sharing between RDZ users and a sensitive receiver. In their framework, when the sensitive user detects interference, it notifies the ZMS that regulates spectrum access in the RDZ. The ZMS restricts access to mitigate interference, progressively reducing RDZ transmitters. Once interference ceases, the ZMS reassigns access, gradually adding more transmitters. The crucial challenge for their reactive approach is quickly determining which RDZ transmitters to revoke or grant access to. In a diverse RDZ with varying usage patterns, their goal is to minimize interruptions to spectrum availability and avoid frequent interference fluctuations at the sensitive receiver. To achieve this, their framework incorporates computing an exponentially weighted moving average (EWMA) of the number of RDZ users operating simultaneously without causing interference. Additionally, they employ weighted variance to capture finer changes in spectrum use, resulting in higher spectrum reuse demonstrated in their results. They present a complete, viable, and deployable solution for spectrum sharing between a sensitive receiver and users within the RDZ.
Rachel Utama (she/her) | Class of 2025 at Northeastern University
▶️ Lyme Disease Biosensing Using Diagnostic Machine Learning Modeling
Supervised machine learning (ML) can be used to support the diagnosis of Lyme disease (LD) using data captured by a grating coupled fluorescent plasmonic (GC-FP) biosensor developed by Ciencia, Inc. The biosensor project aims to create a faster, more sensitive test for Lyme disease than the CDC-recommended two-tiered testing (TTT) system. Potential benefits of using supervised ML to process the biosensor data include more accurate diagnoses than our current standard, a receiver operating characteristic (ROC) threshold test, the ability to match or surpass CDC's original results from the standard TTT test, and additional capabilities such as disease stage classification. GC-FP chips were run with 82 human serum samples representing multiple patient groups, and their respective antibody detection ratios from imaging data were used to train various machine-learning models. The results of these tests were compared against the results from their standard ROC-based test and the original TTT results to determine each algorithm's efficacy. Multiclass Logistic Regression, the highest-performing model thus far, resulted in 95% sensitivity and 93% specificity for this dataset, outperforming results from both the TTT test and the ROC test and thus encouraging future usage of supervised ML as a diagnostic tool for Lyme disease.