The National Center for Women & Information Technology (NCWIT) Selects Recipients of the 2022 NCWIT Collegiate Award
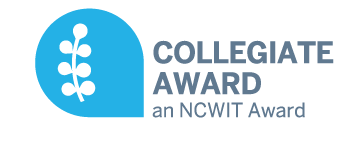
NCWIT is pleased to announce the winners and honorable mentions of the 2022 NCWIT Collegiate Award, honoring the outstanding computing accomplishments of undergraduate and graduate women, genderqueer, or non-binary students. Conferred annually, the award recognizes technical contributions to projects that demonstrate a high level of innovation and potential impact.
Learn about the 2022 recipients by watching the award winning students speak about their projects and what's next for them and by reading about them in the list below.
The entire NCWIT AiC program platform is supported generously by Apple. AiC also receives support for specific national program elements; the NCWIT Collegiate Award is sponsored by Qualcomm and Amazon with additional support from Palo Alto Networks.
2022 Collegiate Award Recipient Videos
- 2022 Freshmen/Sophomore/Two-Year Institution Collegiate Award Recipients
- 2022 Junior/Senior Collegiate Award Recipients
- 2022 Graduate Collegiate Award Recipients
Winners
- Laalitya Acharya, Columbia University, Nereid: Using a Convolutional Neural Network (CNN) Approach, an AI Technique, to Rapidly and Accurately Detect Microbial Contamination That May Cause Water-Borne Diseases
This project developed an affordable, low-maintenance device that can identify contaminants in water and be used to reduce the risk of water-borne disease transmission in areas where people have traditionally lacked access to clean water. The device, called Nereid, employs a microscope camera to take images at regular intervals, and then sends these images to its artificial intelligence processor for analysis. In the event that contamination is identified, the device uses long-range wireless technology to communicate this information to the water plant for further analysis. (View this project online.) - Fatemeh Haghighi, Arizona State University, Transferable Visual Words: Exploiting the Semantics of Anatomical Patterns for Self-Supervised Learning
Computer-aided diagnosis (CAD) systems help physicians better diagnose and treat diseases by using artificial intelligence to more accurately interpret medical images, which have the unique ability to render nuance in internal human anatomy (semantics). This project sought to make CAD systems more efficient by using deep learning to minimize manual labeling efforts in automated image-processing systems. The researcher built a self-supervised learning framework that enables the capture of semantics-enriched information from unlabeled medical image data, enabling CAD systems to detect abnormalities effectively and efficiently using a small number of annotated images. (View this project online.) - Sarah Wielgosz, University of Pittsburgh, Parametric Modeling of NASA's Radioisotope Thermoelectric Generators for Future Deep-Space Missions
Though the National Aeronautics and Space Administration (NASA) has used radioisotope thermoelectric generators (RTGs) to power its probes and rovers for 50 years, the current design methodology does not fully maximize RTG performance. For this project, the researcher worked with the NASA Jet Propulsion Laboratory to design new RTGs capable of completing future deep-space and subsurface exploration missions. Using MATLAB and the university’s supercomputer to develop and test analytic models, a specific power greater than 11 [W/kg] was achieved, more than twice that of current RTGs. (View this project online.)
Honorable Mentions
- Mary Gerhardinger, Kenyon College, The Hubble Tension and Early Dark Energy: Simulating Nonlinear Instabilities
This project builds on the idea that the tension between distinct measurements of the Hubble constant, which indicates how fast the universe is expanding, results from a deficiency in the standard model of cosmology, and it investigates a possible solution by introducing a dynamical scalar field, also known as early dark energy (EDE). EDE “turns on” during the early universe and transfers energy into the universe, thereby changing its expansion. Previous work showed that using a linear analysis of EDE can resolve the Hubble tension. This project focused on demonstrating that a nonlinear model also solves this issue. (View this project online.) - Grace Guan, Stanford University, Early Detection of COVID-19 Outbreaks Using Human Mobility Data
This project leveraged cell phone mobility data to develop models to successfully predict daily new COVID-19 cases and the seven-day test positivity rate for different geographic regions in Israel. This tool can be used to guide government decision making in real time, which is especially important in the post-vaccination era, when focused interventions are needed to contain outbreaks while mitigating the collateral damage from more global restrictions. This prediction model can also be applied to predict outbreaks of other communicable diseases, such as influenza, measles, and SARS. (View this project online.) - Tabitha Edith Lee, Carnegie Mellon University, Structural Sim-to-Real Transfer of Robot Manipulation Policies Using Causal Reasoning
This project is a robot learning methodology called "structural sim-to-real transfer," which looks at how robots can leverage causality to learn how to interact with objects in simulation, and then transfer this learned experience to adapt and learn from new scenarios in the real world. This approach yields robot behaviors that are more compact, more robust to differences between the training and test data, and more efficient to adapt to a real robot than correlation-based approaches. (View this project online.) - Marceline Lewis, Kennesaw State University, Renewable Ocean Wave Energy Harvesting Device for Sustainable Boats and Ships
This project demonstrates that it is possible to efficiently convert the movement of boats and ships caused by wave impacts into a clean renewable energy resource that will make boats more sustainable. Wave energy converters traditionally require direct contact with the water, such as buoys that are designed with a linear generator inside that sways up and down as the buoy rises and falls to generate energy. The project prototype’s mechanism for producing electrical power is designed to sit inside of a boat instead of on the outside, avoiding energy conversion inefficiency resulting from drag. (View this project online.) - Shannon O'Hara Wiora, Tidewater Community College, Comparison of Algorithms for Predicting Membrane Protein Structures
This project involved a comparative analysis of the Rosetta and MODELLER algorithms for homology modeling. Homology modeling is used to predict a protein’s structure. These predictions are important for understanding the protein’s mechanism of action, and they can also be used to study the binding of ligands to that protein, providing a rational and cost-effective platform for drug design. By evaluating the comparative algorithms, the best possible homology models can be developed to evaluate the protein structures, providing a better starting point for understanding protein function and predicting ligand binding poses. (View this project online.) - Trisha Prabhu, Harvard University, ReThink: An Effective Method To Detect and Stop Cyberbullying, Using Context-Sensitive Sentiment Analysis and Deep Learning Algorithms
According to Stopbullying.gov, cyberbullying affects more than 15 percent of U.S. high school students, including more than 55 percent of LGBTQ+ students. This project developed a patented app, ReThink, that can help detect and stop online hate. The ReThink Keyboard is a custom-built QWERTY keyboard that replaces a mobile device’s default keyboard and uses machine learning technology to detect offensive messages. It works across all apps to give users a chance to reconsider sending offensive content. ReThink is currently available in eight languages. (Learn more about this project here and here). - Mehrnoosh Shakarami, University of Texas - San Antonio, Access Control for Smart Home IoT
This project is focused on modeling and implementing mechanisms that appropriately control access to Internet of Things (IoT) systems used in smart home environments, such that only the right people can access the right things at the right time. This includes securing these environments to prevent hackers from gaining control over smart devices even if they are able to get onto the Wi-Fi network, as well as making inter-device communications more automated, increasing comfort and ease of use. (View this project online.) - Christa Simaan, University of Pennsylvania, The Formalization of Permutation Networks
A permutation network uses configurable switches to rearrange the input such that the output is always a permutation of it. Originally developed for telecommunications, these networks are an important part of the Advanced Encryption Standard that powers all web communication technologies. Permutation networks are unique in that they are configurable, reversible, scalable, and parametric polymorphic. This project defined and proved the soundness and completeness of a Benes permutation network using the Coq proof assistant. (View this project online.) - Rachel Sterneck, Yale University, Energy Efficient and Robust Adversary Detection in Neural Networks
Although deep neural networks (DNNs) have great potential to be used in real-world vision tasks, their vulnerability to adversarial attacks – inputs that have been carefully perturbed to fool classifier networks while appearing unchanged to humans – must be addressed to ensure that machine learning applications are safe and reliable. For this project, the researchers created a structured methodology of augmenting a DNN with a detector applied to the most sensitive layer of the network. The method was shown to be an energy-efficient way to improve state-of-the-art detector robustness against adversarial examples. (View this project online.) - Aakriti Upadhyay, University at Albany - SUNY, A Topology-Aware Sampling-Based Motion Planner
Autonomous robotic systems often require pre-planned information about their surroundings to avoid colliding with obstacles when planning their paths. Their configurations contain information on their position, orientation, joint angle, and displacement. However, the topological data extracted (i.e., the connectivity information of the space) by these planners are either not reusable or do not provide much information about the robots’ space. This project introduces a topology-aware sampling-based motion planner that combines the data-extraction features of topological data analysis (TDA) methods with the sampling-based motion planning algorithms to compute an energy-efficient path planner for the robots. (View this project online.) - Sherry Wong, University of Wisconsin - Madison, Cosmic Ray and Magnetically Driven Bubbles in Galaxies
Through a combination of numerical simulations and data-processing methods, this project investigates the behavior of hot, dense gas bubbles accelerated by magnetic reconnection and heated by cosmic rays. How these bubbles form and evolve is significant to understanding how magnetic reconnection can affect the larger galactic system. The researcher found evidence confirming the idea that these bubbles are formed by reconnection, shedding light on the effects of reconnection and the effectiveness of the code that simulates it. (View this project online.) - Rebecca Zhu, Princeton University, MyGraine: Predicting Migraines Using Machine Learning
This project created a tool that uses artificial intelligence to accurately predict migraine occurrences. Existing migraine tracking diaries only allow people to track retrospective information, but having the ability to predict when a migraine will occur can allow them to take preventive measures. Several machine learning and deep learning models were trained and tested using a data set of migraine trigger factors. The trained, best-performing model was integrated into a website called MyGraine. Users can input their migraine triggers and specific indicative features and get predictions on whether they will get a migraine. (View this project online.)